Data Science Driven Investment
Data science combines AI, maths and statistics to provide you investment insights.
Simple is the Best!
​
Our AI models transform complex financial data into simple investment insights.
​
How data science driven investment work?
Data is the heart of AI.
​
Our data scientists walk through comprehensive data science cycle and select AI models for prediction.
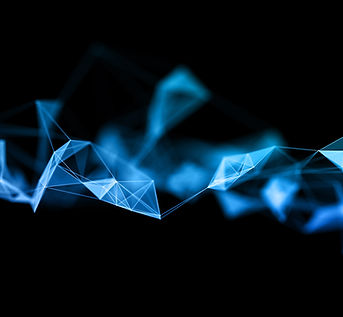
Data Science Cycle
Data Collection
The cycle begins with the data collection. Data can be structured or unstructured from all (relevant) sources, real-time streaming data or historical data. Data science driven approach takes data with an opened mind, data source is not necessary be relevant from our sense. As long as it helps AI models generate more accurate result, we take that data source.
Data pre-processing
Data from data source is not ready to use straight away. Data engineers clean and transform to desired format to fit into the models. This process is most time consuming but essential for ensure data quality standard and impact the model accuracy.
Exploratory Data Analysis (EDA)
EDA is a crucial process to determine the data’s relevance for model building, data scientists conduct this analysis to identify biases, patterns, ranges, and distributions of the data. EDA guides data scientists how to manipulate data sources to identify patterns, detect anomalies, and check model assumptions.
AI Modelling
Data scientists decide which model based on the EDA result and their domain knowledge. Models are trained and tuned until the performance achieved desired accuracy rate. Training models like deep learning models require strong computing power. Performance evaluation is conducted after a model is built. By tuning hyperparameters and adjusting architecture for better prediction.
Explainable Artificial Intelligence (XAI)
XAI is crucial but not common practice in industry so far. Explainable Artificial Intelligence is an approach to designing and developing artificial intelligence systems that can explain their decision-making processes to human users in a way that is understandable and transparent. This approach to AI is seen as a crucial element in ensuring the ethical use of AI technologies, particularly in high-stakes decision-making contexts.
AI Data Intelligence keeps our AI models transparent and explainable. We try our best to explain our methodology, models, and result in easily understandable way.
Data Visualization
AI models serve many purposes in investment, stock prediction, risk prediction and detect market anomality. Forecast results can be communicated by dashboards or reports.